Identifying risk-free customers in a high-risk, negative pincode area?
Is there a safe way to identify risk-free customers in a high-risk, negative pincode area?
High-risk, negative pincode areas have a higher likelihood of fraudulent activity, chargebacks, or other negative events, resulting in increased costs and reduced profits for retailers. Customers from such areas who do not pose a risk of fraud are risk-free customers – valuable and hard to identify. These customers have a good payment history, a stable financial situation, and a low likelihood of returning or canceling an order. In the Indian retail context, identifying risk-free customers is critical for retailers looking to maximize their growth and profitability. By focusing their efforts on serving these customers, retailers can drastically improve their bottom line.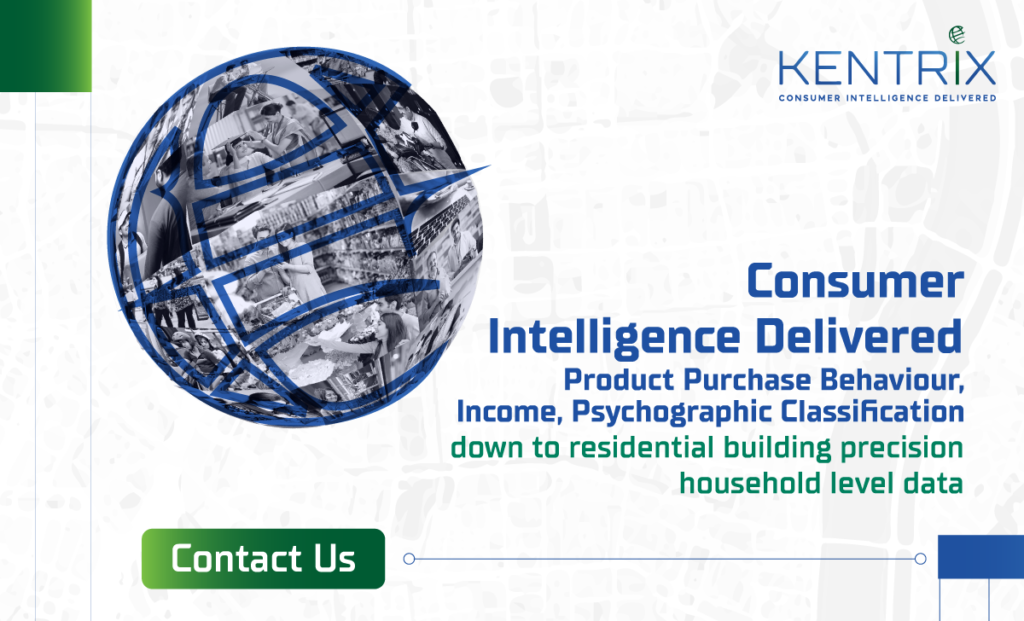
Challenges of Identifying Risk-Free Customers
While identifying these customers is important to enhance the business, the task is not an easy one. The most common challenges faced are:- Data Accuracy and Quality: Retailers often struggle to access accurate and up-to-date information about their customers. This can result in incomplete or outdated customer profiles, making it difficult to accurately determine the risk level of a customer.
- Fraudulent Activity: Fraudulent activity is a common problem in high-risk, negative pincode areas. Retailers need to be able to detect and prevent fraud in real time, but traditional methods of customer identification are often insufficient in identifying potential fraudsters.
- Chargebacks: Chargebacks can have a significant impact on a retailer’s bottom line, especially in high-risk, negative pincode areas. Retailers need to be able to accurately identify customers who are at a higher risk of chargebacks to minimize their financial losses.
- Changing Customer Behavior: Customer behavior is constantly changing, and retailers need to be able to keep up with these changes to accurately identify risk-free customers.
- Limited Resources: Retailers often have limited resources and budgets, making it challenging for them to invest in advanced technologies and solutions for customer identification.
- As a retailer, you need to understand that risk-free customers are not just those who have a good credit history. In high-risk pincode areas, other factors such as demographics, income levels, and spending habits can also play a significant role in determining whether a customer is likely to default on their payments. This is why you need to use big data analytics to collect and analyze data from multiple points and create a comprehensive profile of your customers.
- Using data from social media platforms like Facebook, Twitter, and Instagram is a great way to gather consumer behavior insights to identify risk-free customers. This data can provide valuable information about customers, including their demographics, interests, and spending habits. The information can be used to create a comprehensive consumer profile, giving you a 360-degree view of their financial situation.
- Another way to use big data to identify risk-free customers is by analyzing customer purchase history. By tracking past purchases, you can get a good understanding of customer expenditure behavior and identify those who are likely to be low-risk. This information can be used to create targeted marketing campaigns, customized product recommendations, and more.
- A huge benefit of using big data for customer profiling is the ability to process large amounts of data in real time. This enables you to quickly identify trends and patterns in customer behavior and respond to the changes accordingly by making the necessary adjustments to your offerings.
- Predictive analytics can be used for identification since it uses historical data and algorithms to identify patterns and make predictions about future behavior. In the case of customer risk assessment, predictive analytics can be used to identify those customers who are most likely to default on their payments. By analyzing factors such as customer purchase history, demographics, and spending habits, retailers can create a risk score for each customer and identify those who are low-risk.
- You can implement an early warning system for customer risks. This is a solution that helps you recognize potential risks associated with customers by using AI and ML. By identifying potential red flags, such as suspicious behavior or a history of chargebacks, the early warning system helps you take proactive measures to mitigate risk and make informed decisions about your customer base.
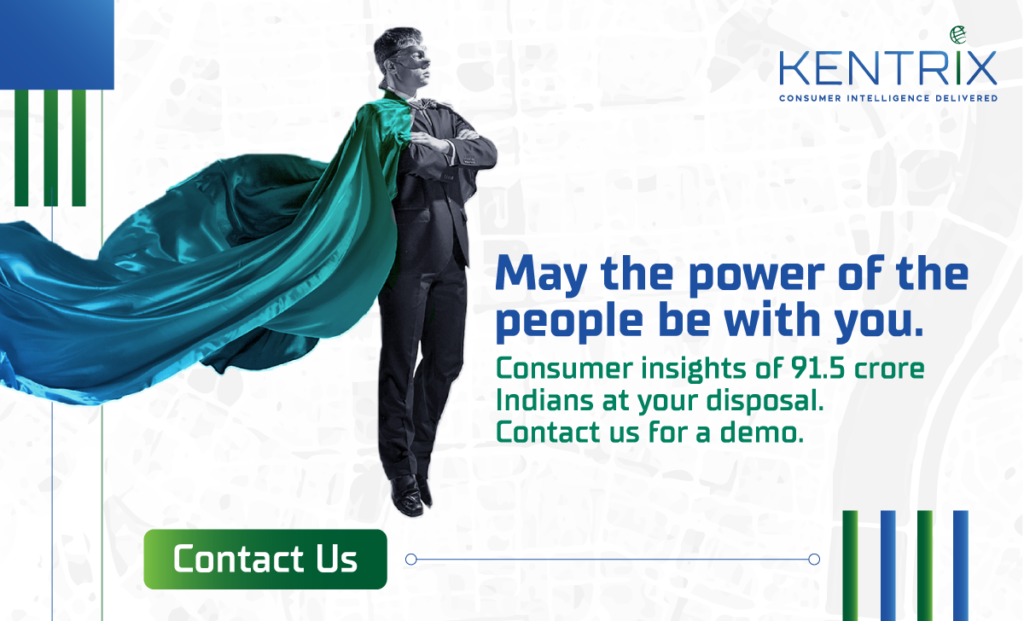
Comments
Post a Comment